- Created by Chelsea Brigg, last modified by Howard Montgomery on Oct 03, 2023
Human-Centered AI: 5 HCD Principles for Developing AI
Reading Time 6 mins
Introduction
In recent years, Artificial Intelligence (AI) has transcended the realms of science fiction to become an integral part of our daily lives. From recommending the next song on your playlist to predicting global financial markets, AI's capabilities are vast, varied, and truly transformative. As with any disruptive technology, the potential of AI is unparalleled, promising solutions to some of the most pressing challenges we face today in healthcare.
Yet, amidst this meteoric rise and potential, lies a pressing concern: For whom is this AI being developed? And more importantly, who gets to decide? This is where the significance of a human-centered approach in AI development emerges. It is not just about building intelligent systems, but about crafting solutions that resonate with, empower, and uplift people, ensuring that technology complements human capabilities rather than competing with or marginalizing them.
Why is Human-Centered AI Critical?
Artificial Intelligence, in all its prowess, is not immune to the complexities of human nature. In fact, when not crafted with a human-centric vision, its impact can be starkly detrimental. Ignoring human factors in AI's development can lead to systems that are not only unhelpful but potentially harmful. Here's why:
- Bias and Discrimination: Without careful consideration, AI can perpetuate, and sometimes amplify, societal biases. These biases, present in data or algorithms, can lead to unfair or discriminatory outcomes, further marginalizing vulnerable populations.
- Loss of Trust: AI systems that act in unpredictable or unsatisfactory ways can quickly lose user trust, making them less likely to be adopted or effectively utilized.
- Safety Concerns: In a critical sector like healthcare, a non-human-centric AI can directly endanger lives due to ill-informed decisions or actions.
Case Study: Oversight in Healthcare AI
IBM's Watson for Oncology was acclaimed as a groundbreaking AI that could help doctors diagnose and treat cancer by providing tailored treatment recommendations based on the analysis of vast medical datasets. However, it faced criticism and challenges upon actual implementation.
In 2018, reports surfaced indicating that Watson had occasionally made unsafe or incorrect treatment recommendations for cancer patients. For instance, there were instances when Watson suggested treatment plans that weren't in line with best practices and clinical guidelines.
A critical issue was how Watson had been trained. Rather than being purely data-driven, it relied heavily on the input from human experts at the Memorial Sloan Kettering Cancer Center. If these experts had a particular bias or if there was a gap in the knowledge provided, Watson might not make a fully informed recommendation.
This situation underscores the importance of the data used to train AI, the potential pitfalls of over-reliance on AI recommendations without human validation, and the challenges of deploying AI in complex, real-world medical scenarios.
5 HCD Principles for Developing AI
As AI becomes increasingly integral to our daily routines, it's vital to create systems that align with human values and needs. Beyond advanced algorithms, the real challenge is ensuring AI resonates and serves its users. Here are five essential HCD principles for genuine human-centered AI development.
- Empathetic Problem Definition: At the heart of effective AI solutions lies a deep understanding of users' genuine needs and emotions. By embracing an empathetic approach, we ensure that AI not only aligns with but also addresses the real challenges faced by users. To truly achieve this, ongoing collaboration with end-users should be woven into every phase of AI strategic planning.
- Fairness and Bias Consideration: Achieving fairness in AI is crucial to avoid amplifying existing societal biases. AI system designers and developers must engage in continual introspection and assessment to prevent inadvertent propagation of these biases. While technical solutions, such as adversarial testing and fairness toolkits, play a pivotal role, a deeper approach demands an appreciation of the broader historical and societal contexts in which these technologies are deployed. As a recommendation, AI development teams should integrate interdisciplinary experts, including sociologists and ethicists, to provide comprehensive insights into the potential socio-cultural implications of AI systems.
- Transparency and Explainability: Imagine boarding a self-driving car that doesn’t tell you how it decides its routes. An unnerving experience, right? This highlights the necessity for AI decisions to be transparent and explainable. Especially in critical domains like healthcare, understanding AI’s reasoning instills trust and facilitates better decision-making. Tools like LIME (Local Interpretable Model-agnostic Explanations) are being developed to demystify the often complex inner workings of AI, enabling users to have a clear view of the decision-making process.
- Privacy and Data Protection: In an age where data breaches make headlines, the importance of user data privacy in AI cannot be overstressed. Every piece of personal information processed by AI systems poses a potential risk if not handled with utmost care, especially in healthcare. There have been instances where seemingly harmless AI applications, like photo-editing apps, were found mishandling user data. Ensuring rigorous data protection protocols, incorporating techniques like differential privacy, and being transparent about data usage are critical steps towards establishing and maintaining user trust.
- Ethical Considerations: Beyond the bits and bytes, AI has profound societal implications. Ethical AI development isn’t just about creating efficient algorithms; it's about asking the deeper questions: Who benefits from the AI? Who might be harmed? Is the AI reinforcing harmful stereotypes or contributing to societal inequalities? Consider the ethical debate around AI in surveillance. While it can enhance security, unchecked usage can lead to invasive privacy violations or state control. Developers need a holistic ethical framework, one that ensures that AI serves humanity and respects fundamental rights.
Case Study: Bridging Gaps in Healthcare AI
Diabetic retinopathy (DR) is the fastest-growing cause of blindness, with nearly 415 million diabetic patients at risk worldwide. Early detection and treatment can significantly reduce the risk, but many patients, especially in low-resource settings, lack access to screening. Recognizing this challenge, Google Health developed a deep learning system to assist eye doctors in identifying DR.
A truly human-centric approach was employed in the design and deployment of this AI solution. It was trained on a dataset of retinal images that were extensively labeled by ophthalmologists, ensuring its foundation was rooted in expert human knowledge. Recognizing the potential diversity of patients, efforts were made to ensure that the training data was representative of various ethnicities and backgrounds.
But what made it particularly human-centered was its usability for the intended end-users: the doctors and medical staff. The AI system provided not just a binary result, but also highlighted the areas of concern on the retinal image, thereby giving physicians a transparent and interpretable result, allowing them to make the final diagnosis.
The result? In field deployments, this AI showed performance on par with U.S. board-certified ophthalmologists. It has been heralded as a potential game-changer for regions where there’s a shortage of ophthalmologists, emphasizing how a human-centric approach to AI can bridge gaps in healthcare and improve patient outcomes.
Conclusion
In the ever-evolving landscape of AI, it's paramount to ensure that our technological advances remain rooted in human needs and values. A human-centric AI not only enhances user trust and satisfaction but also ensures ethical and effective solutions. As we delve deeper into the AI realm, let's prioritize this human-centered approach, making technology an enabler rather than a barrier.
Reference
Gulshan, V., Peng, L., Coram, M., Stumpe, M. C., Wu, D., Narayanaswamy, A., ... & Kim, R. (2016). Development and validation of a deep learning algorithm for detection of diabetic retinopathy in retinal fundus photographs. JAMA, 316(22), 2402-2410.
Ross, C., & Swetlitz, I. (2018). IBM’s Watson supercomputer recommended ‘unsafe and incorrect’ cancer treatments, internal documents show. STAT News.
Continued Learning
Team Essentials for AI: Apply Design Thinking to AI (Free 3-hour training)
https://www.ibm.com/design/thinking/page/courses/AI_Essentials
AI in Healthcare, Standford School of Medicine (5-course specialization via Coursera subscription)
https://www.coursera.org/specializations/ai-healthcare
CHELSEA BRIGG
Chelsea is a Senior Design Strategist with the CCSQ Human-Centered Design Center of Excellence (HCD CoE). For more than a decade she has led mixed-methods user research for science, health, and public policy organizations such as National Geographic, Johns Hopkins Medicine, Penn Medicine, Medicare/Medicaid, and Mathematica Policy Research. Chelsea holds a Master of Science in Human-Centered Computing from the University of Maryland, where she studied as a Computing Research Association scholar.
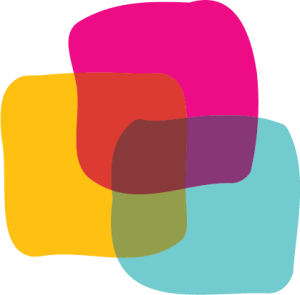
Browse by Topic
-
Page:
-
Blog:
-
Blog:
-
Blog:
-
Blog:
-
Blog:
-
Blog:
-
Blog:
-
Blog:
-
Blog:
-
Blog:
-
Blog:
-
Blog:
-
Blog:
-
Blog:
-
Blog:
-
Blog:
-
Blog:
-
Blog: